Nick Irons
I am a statistician developing (primarily Bayesian) methods to tackle problems in causal inference, model selection and hypothesis testing, nonparametric and high-dimensional statistics, design and analysis of experiments, and modeling of complex data (eg hierarchical, spatiotemporal, mechanistic, and infectious disease models). My work is often motivated by applications in the health and social sciences.
From a methodological perspective, I am interested in expanding Bayesian statistics into new frontiers both through principled modeling and by improving the efficiency and scalability of posterior inference algorithms (eg by incorporating machine learning methods into Bayesian workflow, finding useful parametrisations, or developing sampling algorithms tailored to specific models).
From a modeling perspective, I enjoy drawing on my training in physics to build scientifically-informed models of complex data. I have extensive experience with statistical modeling of data described by differential equations, whether the SIR equations or those of Ornstein-Uhlenbeck, Hamilton, Euler-Lagrange, and Schrödinger. In applied work, I endeavor to provide decision-makers with statistical tools and actionable information by which to make informed choices.
Nick Irons
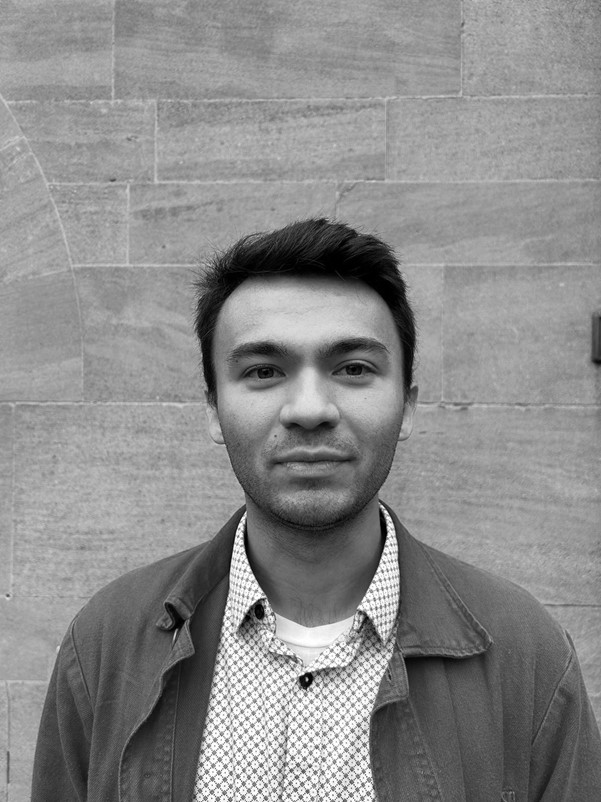
I am a statistician developing (primarily Bayesian) methods to tackle problems in causal inference, model selection and hypothesis testing, nonparametric and high-dimensional statistics, design and analysis of experiments, and modeling of complex data (eg hierarchical, spatiotemporal, mechanistic, and infectious disease models). My work is often motivated by applications in the health and social sciences.
From a methodological perspective, I am interested in expanding Bayesian statistics into new frontiers both through principled modeling and by improving the efficiency and scalability of posterior inference algorithms (eg by incorporating machine learning methods into Bayesian workflow, finding useful parametrisations, or developing sampling algorithms tailored to specific models).
From a modeling perspective, I enjoy drawing on my training in physics to build scientifically-informed models of complex data. I have extensive experience with statistical modeling of data described by differential equations, whether the SIR equations or those of Ornstein-Uhlenbeck, Hamilton, Euler-Lagrange, and Schrödinger. In applied work, I endeavor to provide decision-makers with statistical tools and actionable information by which to make informed choices.